Undergraduate Education about Water and Climate Change: Students’ Use of a Water Balance Model
Lally JSE March 2020 Water Issue PDF
Link to JSE March 2020 Water and Climate Issue Table of Contents
Global climate change is a critical issue affecting both Earth’s climate and water as inextricably paired systems. One of these linkages is found in dwindling groundwater resources. Worldwide, over half of the largest aquifers are over-withdrawn; these areas often overlap with locations of significant surface water stress (United Nations Educational, Scientific and Cultural Organization [UNESCO], 2016). It has been hypothesized that for an increase in global warming of one degree, water availability will be decreased by 20% for nearly 38 million people (UNESCO, 2016). Climate change will exacerbate the unpredictability of weather, which heightens the unreliability of seasonal precipitation for the recharge of freshwater resources (Food and Agriculture Organization of the United Nations & World Water Council, 2015). The complexities of global climate change underscore the importance of fostering individuals’ reasoning about water resources and climate, through formal K-16 classroom settings.
Teaching and Learning about Climate and Water
Climate change and all of its impacts require students to possess accurate conceptions of both Earth’s climate and water systems. Students need opportunities to develop climate literacy (Climate and Energy Awareness Network [CLEAN], 2019), or understanding and abilities to reason and make informed decisions about weather, climate, and its functions and impacts in relation to their environment. Standards for science teaching and learning foreground Earth’s climate, GCC, and water systems as core concepts spanning K-16 learning environments (CLEAN, 2019; USGS et al., 2009), including at the undergraduate level. However, the geosciences are progressively de-emphasized across the K-12 science curriculum (Banilower et al., 2018). Water and climate are topics with which students may engage in a distributed manner in many different course contexts. Water education is critical because of expected changing water availability and profound weather changes in the coming years (Seibert, Uhlenbrook, & Wagener, 2013). At the undergraduate level, most opportunities for students to encounter curriculum surrounding climate change is within the broad discipline of natural resources followed by mathematics and social science (Aubrecht, 2018). This would be adequate if all students at least pursued a minor in one of these fields, however, many students do not take coursework in these content areas during their time in college. Course descriptions feature climate change in as little as 10% of core curriculum courses (general education courses) while students have a 17% chance of enrolling in a minimum of one climate change focused coursed throughout their core curriculum (Hess & Collins, 2018).
Perhaps as a result, undergraduate students possess scientifically inaccurate ideas about water (Halvorson & Westcoat, 2002) and climate (Libarkin et al., 2015), with these alternative understandings persisting into adult, post-educational life (Abbott et al., 2019; Duda et al., 2005). Students specifically struggle with water related concepts such as evaporation and latent heat (Cardak, 2009) and climate related concepts such as the impact of climate change on the ozone layer (Libarkin et al., 2015). These types of inaccurate ideas reflect a rudimentary understanding to which, linear mono-causal thinking contributes. This type of thinking is difficult to overcome because it implies a direct cause and effect relationship for processes that are in reality much more complex (Raia, 2008). Students need to be able to conceptualize the water cycle, but they must also know how resources and living things interact through various cycles (NGSS Lead States, 2013). In light of the persistent learning gaps, students need more and more effective opportunities to encounter climate change and water curriculum in order to learn to reason about climate and its related components (Abbott et al., 2019).
Scientific Models and Modeling in Undergraduate Education
Models are a tool that instructors can use to help students learn to reason more effectively about climate and water systems. Computer-based models can be used to provide a visual representation of what students would otherwise perceive as invisible, such as climate, its change over time, or groundwater movement. Models, when used in parallel with a suite of other active-learning strategies, can contribute to student learning surrounding climate and water through hypothesis experimentation (Lally & Forbes, 2019) and the ability to visualize system patterns (Carey & Gougis, 2017). Computer based-water models rely on the user to input accurate information in order to receive an output from which they can make a decision. Students must know most or all of the interacting components and how the data presented from a model will affect or be affected by such interactions and components. Models are only useful in decision making if the learner can make use of the graphic output and apply it to a situation with the inclusion of the most recent theories and data as well as current interactions between components. There have been calls for increased implementation of climate and water model use in undergraduate courses, including practices such as science-based teaching strategies and computational modeling (CUAHSI, 2018; Mosher et al., 2014). Unfortunately, neither climate models nor water models are frequently used in introductory undergraduate coursework (Merwade & Ruddell, 2012; Tasquier et al., 2016), reinforcing a limited emphasis on modeling in undergraduate geoscience courses (Lally et al., 2019).
Regardless of the disciplinary focus of students’ investigation, modeling involves a set of core modeling practices including model development, prediction, questioning, explanation, evaluation, revision, and support of ideas (Forbes et al., 2015) and the epistemic dimensions of representation, evidence, and explanation (Lally & Forbes, 2019). Of these modeling practices and epistemic dimensions, model use and evaluation are just two of the skill sets students learn using models in the course. Model use in this context includes the students’ participation in modeling habits consisting of using the model to make a hypothesis, determine relationships between variables, and citing the model as evidence to substantiate claims (Lally & Forbes, 2019). Evaluation of a model comprises skills including modification, contrasting, validating the accuracy and precision (Coll et al., 2005; Gouvea & Passmore, 2017) and suitability for a context (Pluta, Chinn, & Duncan, 2011). The ability to evaluate a model contributes to enhanced model-based reasoning (Gobert & Buckley, 2000). Model organization and power can fluctuate from model to model; students require opportunities to interact with different models to practice the skills of use and evaluation in different contexts.
All models, including computer-based models of water systems, are limited in their scale and scope (Habib, Ma, Williams, Sharif, & Hossain, 2012) and range in accuracy and contextual fit (Lally & Forbes, 2019). Therefore, students need opportunities to practice using several different types of models in order to evaluate them successfully (Lally & Forbes, 2019). Yet, more opportunities for simulation modeling, interaction with authentic data, and the application of other active learning opportunities are needed to support hydrologic courses and learning (CUAHSI, 2010; Merwade & Ruddell, 2012; Ruddell & Wagener, 2014). To begin to address this need, we developed and studied the implementation of a computer-based water simulation model in an undergraduate water course.
Supporting Students’ Model-Based Learning about the Water Balance: A Case Study
Here, we report on the use of a computer-based water modeling tool developed for an interdisciplinary, introductory-level course, Water in Society (Forbes et al., 2018), which serves both STEM and non-STEM majors. After learning to use the model, students completed a decision making task which was justified using model outputs as evidence. In a previous study, we found students’ evaluation of the WBM improved from year 1 to year 2 surrounding themes of model complexity, generalizability, and specificity (Lally & Forbes, 2019). Here, we investigate quantitative results surrounding gender, year, and year of a computer-based simulation water model assignment and qualitative findings of student reasoning on the effect of precipitation and potential evapotranspiration on the water table. This work is part of our team’s broader research program focused on teaching and learning about water across the K-16 continuum (Forbes et al., 2018; 2015; Lally & Forbes, 2019; Owens et al., under review; Petitt & Forbes, 2019; Sabel et al., 2017).
Course Context
Students were enrolled in an introductory, interdisciplinary, water course, SCIL 109: Water in Society at a large Midwestern University. The course, offered every spring beginning in 2017, meets twice as a whole class and once for a one-hour, small-group lab weekly, for a total of 3-credit-hours (Forbes et al., 2018). Contributing to the interdisciplinary nature of the course, three faculty members from different disciplines- agricultural economics, a hydrogeophysicist, and science education, along with two graduate students, were part of the developmental and instructional team for the course. The course incorporates increasing interconnectivity of the FEW-Nexus and projects to support course content spanning multiple weeks (Lally & Forbes, 2019). Integrated within the course are both the human and natural aspects of systems (i.e. socio-hydrologic systems). Students represented a variety of STEM and non-STEM majors due to the course fulfillment of several general education requirements for the University (Table 1). Students were evenly distributed between genders and included a large proportion of study-abroad students from Africa and Asia. Instructors, course goals, and assessments were the same in each iteration of the course (Lally & Forbes, 2019).
Table 1 | ||||||||||
Student Demographics from 2017, 2018, 2019 | ||||||||||
Female | Male | Freshmen | Sophomore | Junior | Senior/+ | STEM Major | Non-STEM Major | |||
2017 | 15 | 20 | 9 | 10 | 9 | 7 | 26 | 9 | ||
2018 | 27 | 21 | 2 | 24 | 13 | 9 | 44 | 4 | ||
2019 | 19 | 27 | 5 | 16 | 16 | 9 | 42 | 4 |
Outside of class, students were responsible for learning content through readings, videos, simulations, and worksheets. During class, students practiced and refined their ideas about content through large and small group discussions, small group activities, worksheets, and group decision making. Throughout the course, students created and revised an infographic about a water-related issue, completed summative assignments surrounding two computer-based water models, and explored a regionally relevant sociohydrologic issue through systems thinking as a capstone assessment.
Water Balance Model (WBM)
The Water Balance Model (WBM) is an online modeling tool that allows the student to simulate realistic future scenarios investigating the tradeoffs between land use (i.e. irrigation intensity) and water table decline across four climate zones within the state of Nebraska (Fig 1). Because of the coupled nature of surface and groundwater, this problem is particularly challenging for both policy making as well as developing realistic water balance simulation tools.
The WBM simulates a 1-dimensional bucket type water balance using:
Where P is the yearly precipitation (mm/yr), I is the yearly irrigation (mm/yr), ET is the yearly evapotranspiration (mm/yr), Q is the streamflow (mm/yr), S is the change in unconfined aquifer storage (mm), and t is time (yr). In order to simulate the future streamflow and water table change the student specifies a number of inputs in the Graphical User Interface (Fig. 2). These include: the number of years to simulate (range 5-125), the climate zone (1-4), the runoff ratio (fraction of rainfall that is assumed to directly turn into streamflow, 0-1), fractional cover of irrigated corn and irrigated soybean (remainder is natural grassland vegetation and all three terms must sum to 1). Following the scenario selection, P and potential ET (PET) are generated stochastically for each month using the long-term historical data (Sharma & Irmak, 2012 a;b; Wang & Zlotnik, 2012). The monthly totals are then summed to determine both growing season (May through September) and annual totals. Next Q is calculated directly from the runoff ratio input set by the user. Note this runoff ratio and the fraction of irrigated area are the key “knobs” to tune the model output to meet the criteria of the scenario and justify the policy decision. Using the simulated PET and P, ET and I for the corn, soybean, and native grassland (I = 0) areas can now be calculated. Finally Eq. (1) and the estimates of the individual fluxes by land use (e.g. P, I, ET, Q), the change in aquifer storage and thus water table decline can be calculated (see Fig. 2 for example solution). Advanced climate options allow the student to change the future pattern of rainfall and potential ET (e.g. inflation and deflation factors of historical annual averages) such that scenarios can mimic output and predictions from General Circulation Models (Pachauri et al., 2014).
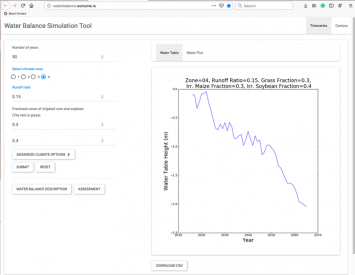
Figure 2. Graphical user interface the student uses to simulate water balance scenarios. Here a scenario was selected and the graphical results are displayed.
The Water Balance Model allowed students to model the effects of a changing climate and land use on groundwater resources in Nebraska. Students then used this scenario data to make a decision about groundwater use in the face of an unpredictable climate. In the context of the course, students used the WBM to explore climate and hydrologic scenarios that would be otherwise impossible without the use of a model. For example, students use the WBM to test different advanced climate options including P or PET annual mean inflation/deflation, variance inflation/deflation, and net irrigation requirement reduction for either corn or soybeans. Using the resulting graphical outputs, in small groups, students practice making decisions surrounding the quantity of irrigated acres that would result in a stable water table (defined in the class as a change of less than +/- 1m over 100 years). Students can also use the WBM to investigate runoff ratio ramification for both change in water table height and annual water table decline. This information allows students to test the predicted severity of changes in farming and climate on groundwater availability and concomitant changes in streamflow. We note that in climate zone 1 land economic assessments between irrigated and rainfed areas differ by a factor of ~4, with center pivot irrigated crop being assessed at $2700/acre and rainfed being evaluated at $700/acre in 2018 for Northwest Nebraska (Jansen & Stokes, 2018) directly affecting the rural economy and livelihood of stakeholders. As an advanced climate option, the students are able to change how rainfall and temperature/PET may be affected in the future (compared to the historical average) and how that might affect the sustainability of the system. Outputs from the model are both a graphical solution (Fig. 2) and CSV of the yearly simulations for further analysis.
The WBM Project
Students completed a summative assignment surrounding the WBM in which they answered a series of questions and completed exercises that looked at the sustainability of the overall agricultural production, groundwater, and surface water systems. Here the sustainability of the system is impacted by the tradeoffs between reduced streamflow, water table decline, and fractional area used for irrigation agriculture (i.e. economic livelihood of local stakeholders). The students imagined themselves as a water manager of a Natural Resources District in Nebraska and make justifiable decisions about fractional land use that balanced the needs of various stakeholders including environmentalists and producers. Additionally, students learned to evaluate the WBM through explicit discussions about its limitations, utility for decision making despite its limitations, and potential WBM improvements. The WBM contains climate and groundwater components to evaluate, giving students the opportunity to compare two types of information between and within models.
Part I. In Part 1 of the assignment, students selected a climate zone (Figure 1) and identified the runoff ratio resulting in a stable water table over the next 100 years with 100% grass cover. Next, they answered set of questions building off their initial runoff ratio finding. Students were asked to find the runoff ratio resulting in a stable water table for the following scenarios: 10% irrigated corn, 10% irrigated soybean, 25% irrigated corn, 25% irrigated soybean,
50% irrigated corn, and 50% irrigated soybean. Responses were accompanied by a brief description of each graph in terms of production and water table maintenance (Figure 3).
Figure 3. Graphical user interface example of predicted water table height change for a 75 years period in Zone 3 of Nebraska with 10% runoff ratio and fractional land cover of 20% irrigated corn, 17% irrigated soybean, and 63% grass cover.
Using the graphical outputs and descriptions, students then made a decision concerning the number of acres they would allocate for corn and soybean production in their Natural Resource District, described the impact of their decision on runoff ratio and streamflow, and justified their decision to the Natural Resources District Board of directors including multiple stakeholders. In this way, students demonstrated their ability to operate the model, interpret the outputs, and apply the predicted outcomes to real-world problems.
Part 2. Part 2 of the WBM assignment required students to use the same Zone as Part 1, but in the context of advanced climate options following hypothetical outputs from general circulation model emission scenarios affecting changes in precipitation and air temperature/PET (Figure 4). Students used the model to make contour graphs and identify, for 100% grass cover, what runoff ratio gives a stable water table for rainfall mean inflation/deflation factors: 0.6, 0.8, 1.2, and 1.4. A graphical solution was required for the answer with a label for the runoff ratio of each. Students wrote a summary of their graphical and runoff ratio findings including ideas such as the effect of rainfall on production and the water table.

Figure 4. Graphical user interface example of expected annual water table decline for a 75-year period in Zone 3 of Nebraska with a rainfall mean inflation/deflation factor of 60%.
For the second question set of Part 2, students used the contour tab to find, for 100% grass cover, the runoff ratio that gives a stable water table for the PET mean inflation/deflation factors of: 0.6, 0.8, 1.2, 1.4. A complete response included a graphical solution with the runoff ratio labelled. This information was used to generate a paragraph summary of their findings from this set of graphs including ideas such as the effect of PET on production and the water table.
As a synthesis question, students were asked, “What has a larger effect on the water table for the same magnitude of change, a change in mean precipitation or mean PET?”
In the last question of Part 2, students also revisited the decision they made in Part 1 about the fraction of irrigated acres they would permit in their Natural Resources District. They are instructed to think about the effects of climate change on water resources in the future in reference to their decision. Answers from Part 1 and 2 could be used to defend their decision or to change their decision based on anticipated climate effects. For reference, three key figures on GCM output were provided to help guide their decision and provide justification to the board on the level of climate risk they were willing to consider. Some students changed their percentage of irrigated acres while others did not, each response was defended using graphical output from the model to predict future effects of climate change on production, streamflow, and runoff ratio.
Part 3 of the assignment was a reflection on the Water Balance Model. Students reflected on the overall strengths and weaknesses of the WBM, any information they needed to help make decisions as an NRD manager, and the general benefits of modeling.
Results
Findings from the data analysis of student work reveal consistencies across student demographics from multiple years of the course. Qualitative results reveal encouraging comparative trends overall in student reasoning surrounding the WBM. Overall, exploring climate and water relationships through the WBM presents students with an opportunity to revise their thinking about water resources and the Food-Energy-Water-Nexus. Through analysis of the WBM summative assessment, we were able to determine that there is no difference between gender, academic year, or major (STEM or non-STEM) in students’ ability reason about the WBM model (Figure 2 and 3). These findings suggest that because of the active learning methods employed in the course, students of all backgrounds, years in college, and genders can effectively engage with the WBM to explore groundwater and climate variables.
Table 2 | |||||||
t-test Results of WBM Scores by Gender, and Major | |||||||
n | Mean | SD | df | t | p | d | |
Male | 73 | 0.73 | 0.16 | 136 | 0.56 | >.05 | 0.06 |
Female | 65 | 0.74 | 0.18 | ||||
STEM | 123 | 0.74 | 0.17 | 36 | 1.79 | >.05 | 0.32 |
Non-STEM | 15 | 0.68 | 0.2 |
Table 3 | |||||
One-Way Analysis of WBM Scores by Year | |||||
df | SS | MS | F | p | |
Between groups | 3 | 0.02 | 0.008 | 2.67 | >.05 |
Within groups | 134 | 3.92 | 0.03 | ||
Total | 137 | 3.94 |
However, results of qualitative analysis show that students struggled with the ability to discern the difference between the effect of precipitation and PET on the water table. Specifically, they struggled to identify which variable, precipitation or PET, had a larger effect on water table height for the same magnitude of change; compounded by the difficulty students had in reading contour graphs. This obstacle was consistent across course iterations, regardless of whether WBM reasoning improvement increased. For example, one student wrote, “I don’t see a significant difference between the two when looking at runoff ratios. At the same values for deflation and inflation you get roughly the same runoff.” 219_WBM. This student did not describe the difference observed between PET and rainfall on the water table. This may be a product of the lack of specificity in model outputs as well. For example, the outputs do not have a finer grain than 10% for runoff ratio. This makes comparing runoff ratios between scenarios difficult because the values look similar on the graphs, but could be larger if the runoff ratio scale were finer. Another student responded to the question about water table effect by writing, “I think that both are equally important, depending on the area, year, etc.” 158_WBM. This student identified that year and area are important variable when considering the impact of PET and rainfall inflation/deflation on water table height, but did not identify which had a greater effect on the assignment outputs. The ability to effectively read contour plots has a significant impact on a student’s ability to determine quantitative differences between variables.
Course Outcomes and Next Steps
Conducting research in iterative offerings of the same course has allowed the instructional team to make changes between years based on student feedback and statistical analysis of student work (Forbes et al., 2018; Lally & Forbes, 2019). Overall, we have moved towards a flipped-style course structure increasingly over time to afford students better ways of working with the WBM. A flipped course context offers students time to think about content outside of class, distributing their learning (Gross et al., 2015), while in class time is devoted to practicing the content through discussions, questioning, and evaluating (Jones et al., 2019; Zainuddin & Perera, 2019). In the Water in Society course, course content related to the WBM is now presented fully online so that students can begin to practice using the model on their own, then come to class ready to start working in groups and answering questions. Class meetings are designed to be student-centered, utilizing an array of active learning strategies. For example, carefully selecting activities and discussion questions surrounding modeling has shown to help increase learning gain in model use and evaluation (Lally & Forbes, 2019). Findings presented here suggest that because of the active learning methods employed in the course, students of all backgrounds, years in college, and genders can effectively engage with the WBM to explore groundwater and climate variables.
Moving forward, due to the interdisciplinary nature of the course and the variety of content, more focus needs to be placed on the connection between the energy, water, and economic components of the course. Students are exposed to the connection between food and water several times throughout the course through models, guest speakers, and even their own personal experiences using water to grow food. Yet, the link between economy and sustainability needs to be more explicit. This could take the form of incorporating an economic component to the WBM to make it more robust. For example, adding in the costs for yield differences between irrigated and rainfed acres, as well as costs for the pumping of water depth below the surface increases. Another factor to include could be the difference in land valuations of irrigated versus rainfed acres as a direct impact on the tax base for the state. This would have huge ramifications on school funding and infrastructure, which could further exasperate the urban and rural conflict (i.e. sociohydrolgoy). The increasingly unpredictable nature of climate events directly relates to choices made over the past decades. Therefore, it is critical that students experience models such as the WBM, which allow them to evaluate water needs for a variety of users in the context of a rapidly changing climate. Fully supporting students in learning the interrelated facets of sociohydrologic and climate issues is at the heart of this course. In the future, a premium will continue to be placed on students’ use of models to explore the ways human and natural systems interact.
Data availability
The WBM online simulation tool is freely available at: http://waterbalance.outcome.io/. Model code, description, and assessment are available upon request.
References
Abbott, B. W., Bishop, K., Zarnetske, J. P., Minaudo, C., Chapin, F. S., Krause, S., … & Plont, S. (2019). Human domination of the global water cycle absent from depictions and perceptions. Nature Geoscience, 12, 533–540.
Aubrecht, K. B. (2018). Teaching relevant climate change topics in undergraduate chemistry courses: Motivations, student misconceptions, and resources. Current Opinion in Green and Sustainable Chemistry, 13, 44-49.
Banilower, E. R., Smith, P. S., Malzahn, K. A., Plumley, C. L., Gordon, E. M., & Hayes, M. L. (2018). Report of the 2018 NSSME+.
Cardak, O. (2009). Science students’ misconceptions of the water cycle according to their drawings. Journal of Applied Sciences, 9(5), 865-873.
Carey, C. C., & Gougis, R. D. (2017). Simulation modeling of lakes in undergraduate and graduate classrooms increases comprehension of climate change concepts and experience with computational tools. Journal of Science Education and Technology, 26(1), 1-11.
Climate and Energy Awareness Network (CLEAN). 2019. Available at http://cleanet.org/ (accessed 20 November 2019).
Coll, R. K., France, B., & Taylor, I. (2005). The role of models/and analogies in science education: Implications from research. International Journal of Science Education, 27(2), 183-198.
Consortium of Universities for the Advancement of Hydrologic Science, Inc. (CUAHSI) (2018). Water in a dynamic planet: A five-year strategic plan for water science, CUAHSI, Washington, D.C.
Duda, M. D., De Michele, P. E., Jones, M., Criscione, A., Craun, C., Winegord, T….Herrick, J. B. (2005) Americans’ knowledge of and attitudes toward water and water-related issues. Harrisonburg, VA: Responsive Management.
Food and Agriculture Organization of the United Nations & World Water Council. (2015). Towards a water and food secure future. Critical perspectives for policy-makers.
Forbes, C. T., Brozovic, N., Franz, T., Lally, D., & Petitt, D. (2018). Water in Society: An interdisciplinary course to support undergraduate students’ water literacy. Journal of College Science Teaching, 48(1), 36-42.
Forbes, C., Zangori, L., Vo, T., & Schwarz, C. (2015). Supporting students’ scientific modeling when learning about the water cycle. Science and Children, 53(2), 42-49.
Gobert, J. D., & Buckley, B. C. (2000). Introduction to model-based teaching and learning in science education. International Journal of Science Education, 22(9), 891-894.
Gouvea, J., & Passmore, C. (2017). Models of ‘versus’ models for. Science & Education, 26(1-2), 49-63.
Gross, D., Pietri, E. S., Anderson, G., Moyano-Camihort, K., & Graham, M. J. (2015). Increased preclass preparation underlies student outcome improvement in the flipped classroom. CBE—Life Sciences Education, 14(4), ar36.
Habib, E., Ma, Y., Williams, D., Sharif, H. O., & Hossain, F. (2012). HydroViz: Design and evaluation of a Web-based tool for improving hydrology education. Hydrology and Earth System Sciences, 16(10), 3767-3781.
Halvorson, S. J., & Westcoat, J. L., Jr. (2002). Problem-based inquiry on world water problems in large undergraduate classes. Journal of Geography, 101(3), 91–102.
Hess, D. J., & Collins, B. M. (2018). Climate change and higher education: Assessing factors that affect curriculum requirements. Journal of Cleaner Production, 170, 1451-1458.
Jansen, J., & Stokes, J. (2018). Nebraska farm real estate market highlights 2017-2018. Nebraska Farm Real Estate Reports, 45, 1-56
Jones, J. P., McConnell, D. A., Wiggen, J. L., & Bedward, J. (2019). Effects of classroom “flipping” on content mastery and student confidence in an introductory physical geology course. Journal of Geoscience Education, 1-16.
Lally, D., & Forbes, C. T. (2019). Modeling water systems in an introductory undergraduate course: Students’ use and evaluation of data-driven, computer-based models. International Journal of Science Education, 41(14), 1999-2023.
Libarkin, J. C., Thomas, S. R., & Ording, G. (2015). Factor analysis of drawings: Application to college student models of the greenhouse effect. International Journal of Science Education, 37(13), 2214-2236.
Merwade, V., & Ruddell, B. L. (2012). Moving university hydrology education forward with geoinformatics, data and modeling approaches. Hydrology & Earth System Sciences Discussions, 9(2). 2601-2628.
Mosher, S., Bralower, T., Huntoon, J., Lea, P., McConnell, D., Miller, K., Ryan, J., Summa, L., Villalobos, J., & White, L. (2014). Future of undergraduate geoscience education: Summary report for summit on future of undergraduate geoscience education.
NGSS Lead States (2013). Next generation science standards. For states, by states. Washington, DC: The National Academies Press.
Owens, D., Pettit, D. Lally, D., & Forbes, C.T. (under review). Undergraduate students’ reasoning about socio-hydrologic challenges: Scientific literacy and informed decision-making in interdisciplinary STEM. In Research in Science Education.
Pachauri, R. K., Allen, M. R., Barros, V. R., Broome, J., Cramer, W., Christ, R., … & Dubash, N. K. (2014). Climate change 2014: Synthesis report. Contribution of Working Groups I, II and III to the fifth assessment report of the Intergovernmental Panel on Climate Change (p. 151). IPCC.
Petitt, D.N. & Forbes, C.T. (2019). Values use of undergraduate students in socio-hydrological reasoning: A comparative study. Natural Sciences Education, 48(1), 1-12.
Pluta, W. J., Chinn, C. A., & Duncan, R. G. (2011). Learners’ epistemic criteria for good scientific models. Journal of Research in Science Teaching, 48(5), 486-511.
Raia, F. (2008). Causality in complex dynamic systems: A challenge in earth systems science education. Journal of Geoscience Education, 56(1), 81–94.
Ruddell, B. L., & Wagener, T. (2013). Grand challenges for hydrology education in the 21st century. Journal of Hydrologic Engineering, 20(1), A4014001-1-8.
Sabel, J.L., Vo, T., Alred, A., Dauer, J.M., & Forbes, C.T. (2017). Undergraduate students’ scientifically-informed decision-making about socio-hydrological issues. Journal of College Science Teaching, 46(6), 64-72.
Seibert, J., Uhlenbrook, S., & Wagener, T. (2013). Preface “Hydrology education in a changing world”. Hydrology and Earth System Sciences, 17(4), 1393-1399.
Sharma, V., & Irmak, S. (2012 a). Mapping spatially interpolated precipitation, reference evapotranspiration, actual crop evapotranspiration, and net irrigation requirements in Nebraska: Part I. Precipitation and reference evapotranspiration. Transactions of the ASABE, 55(3), 907-921.
Sharma, V., & Irmak, S. (2012 b). Mapping spatially interpolated precipitation, reference evapotranspiration, actual crop evapotranspiration, and net irrigation requirements in Nebraska: Part II. Actual crop evapotranspiration and net irrigation requirements. Transactions of the ASABE, 55(3), 923-936.
Tasquier, G., Levrini, O., & Dillon, J. (2016). Exploring students’ epistemological knowledge of models and modelling in science: Results from a teaching/learning experience on climate change. International Journal of Science Education, 38(4), 539-563.
United Nations Educational, Scientific and Cultural Organization. (2016). The United Nations world water development report 2016 “water and jobs”.
USGS, U. Army Corps of Engineers, US Department of the Interior, and NOAA. 2009. Climate change and water resources management: a federal perspective. Reston, Va.
Wang, T. J., & Zlotnik, V. A. (2012). A complementary relationship between actual and potential evapotranspiration and soil effects. Journal of Hydrology, 456, 146-150.
Zainuddin, Z., & Perera, C. J. (2019). Exploring students’ competence, autonomy and relatedness in the flipped classroom pedagogical model. Journal of Further and Higher Education, 43(1), 115-126.